#eCommerce App Data Scraping
Explore tagged Tumblr posts
Text
eCommerce App Data Scraping | Retail Data Extraction Services
Gain a competitive edge with our eCommerce and retail data scraping services in the USA, UK, UAE, India, China, and Spain. Unlock growth opportunities today.
know more: https://www.mobileappscraping.com/ecommerce-app-scraping-services.php
1 note
·
View note
Text
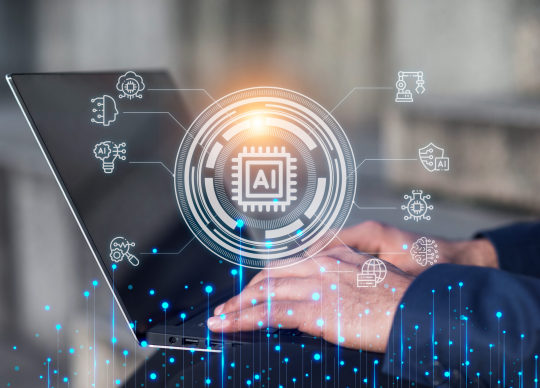
Unlock Ecommerce Success with Codeperk Solutions
1 note
·
View note
Text
7 Competitive Intelligence Benefits for e-Commerce Business

E-commerce businesses are used to gathering and analyzing data. This is one of the reasons why they are willing to pay more for analytics software than others.
But what about competitors? How do you know if you are doing better than your rivals? Gathering competitive intelligence is the key to boosting sellers' ROI. It helps you understand your customers, competitors, and the marketplace as a whole. It is a meaningful way to stay one step ahead of the competition.
What Is Competitive Intelligence In E-Commerce?
Competitive intelligence is collecting and using information about your competition to your advantage. It might sound like spying, but it is a prevalent practice used by all companies — even yours!
You can learn from your competitors' successes and mistakes. If you know what they are doing right, you can replicate their success in your own business. And if they're making mistakes, you can avoid them. If you are not already using competitive intelligence, it's time to start. Here are a few reasons why.
#Competitive intelligence#eCommerce Data Scraping#Competitive Pricing Intelligence#web data scraping#mobile app scraping#ecommerce web scraping tool#Data Scraping Services
0 notes
Text
Scrape Popular Ecommerce App Data
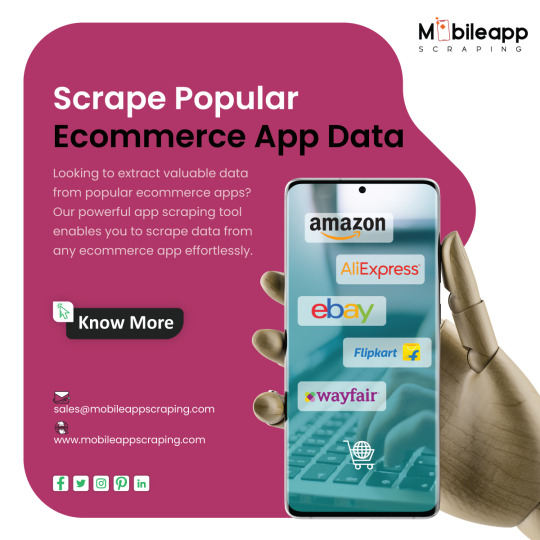
Looking to extract valuable data from popular ecommerce apps? Our powerful app scraping tool enables you to scrape data from any ecommerce app effortlessly.
#scrape eCommerce app data#extract data from eCommerce app#eCommerce app scraping services#popular eCommerce app scraping services
0 notes
Text
Global Guide to Web Scraping Wine Pricing Trends
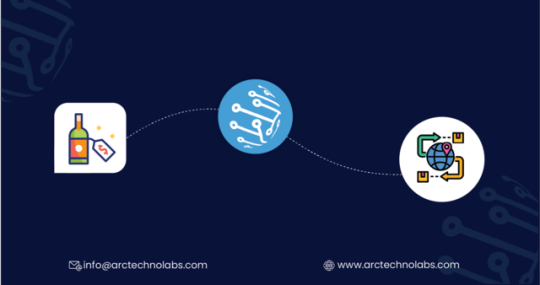
Introduction
In an era where consumer preferences and global trade dynamics shift rapidly, understanding real-time wine pricing trends is crucial for businesses in the alcohol industry. Web scraping wine pricing trends has become a powerful solution for importers, distributors, sommeliers, and wine retailers seeking a data-driven edge in global markets.
By using advanced tools to Scrape Alcohol Price Data Intelligence, professionals can access updated price listings, market fluctuations, regional demand patterns, and promotional pricing—helping them make smarter procurement and pricing decisions.
The Role of Data in the Wine Industry
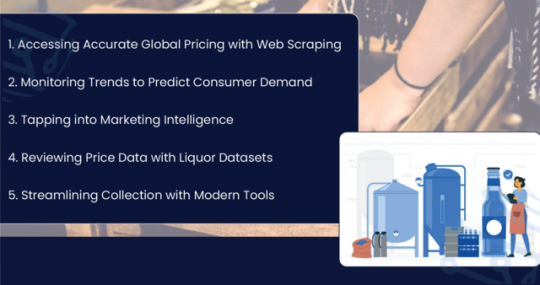
The global wine industry is driven by intricate factors—vintage, region, availability, and seasonal consumption—that impact pricing and demand. As the market becomes more competitive and digitalized, businesses can no longer afford to rely on outdated reports or manual tracking. Instead, they are turning to Web Scraping Services and intelligent tools to gather real-time insights, allowing them to stay agile and data-informed in a fluctuating industry.
1. Accessing Accurate Global Pricing with Web Scraping
One of the biggest advantages of using Web scraping wine pricing trends is the ability to collect pricing data across various geographies. Importers, distributors, and sommeliers can monitor price shifts for specific varietals in real time.
Example Stats: Average Retail Wine Prices (2020–2025)
2. Monitoring Trends to Predict Consumer Demand
By using a Wine industry data scraper tool, businesses can Analyze wine sales trends 2025 to predict seasonal demand patterns and adjust inventories accordingly.
Example Stats: Seasonal Sales Growth (%) by Quarter (2020–2025)
3. Tapping into Marketing Intelligence
Advanced scrapers can Extract wine marketing data such as promotional offers, product descriptions, and regional campaigns from online retailers. This can be especially helpful for crafting hyper-targeted campaigns.
Example: Marketing Mentions by Region (2025)
4. Reviewing Price Data with Liquor Datasets
With access to Liquor or Alcohol Price Review Datasets , stakeholders can monitor long-term price trends, promotional impacts, and regional differentials to plan bulk orders or adjust their retail pricing strategies.
Example: Price Variation for Red Wines (2020–2025)
5. Streamlining Collection with Modern Tools
Businesses now employ Web Scraping Liquor or Alcohol Data technologies such as Web Scraping API Services and Mobile App Scraping Services to automatically Scrape wine sales data 2025 and retrieve structured insights without maintaining manual workflows.
Using these tools, companies can Scrape Alcohol Price Data Intelligence directly from marketplaces, apps, and eCommerce stores in real time—enhancing agility and responsiveness.
By investing in smart solutions like Web scraping wine pricing trends, companies gain a competitive advantage through accurate forecasting, better marketing strategies, and optimized purchasing. Data is no longer optional in the wine trade—it's your most valuable asset.
Key Benefits of Web Scraping Wine Pricing Trends

1. Global Price Benchmarking
With Web scraping wine pricing trends, importers can benchmark prices across countries and retailers. This helps in identifying profitable sourcing regions and preventing overpricing in local markets.
Example:
2. Sales Forecasting and Demand Planning
Distributors can Analyze wine sales trends 2025 by combining pricing data with seasonal sales activity. This helps forecast stock requirements and optimize distribution.
Example:
3. Retailer and Competitor Analysis
Using a Wine industry data scraper tool, sommeliers and retailers can monitor top-performing SKUs, limited-edition releases, and pricing strategies of competitors.
Example:
4. Extracting Marketing Insights
Professionals can Extract wine marketing data from promotional banners, discount listings, and regional campaigns. This intelligence can guide more effective marketing and ad targeting.
5. Mobile App Data Insights
Many wine retailers now offer mobile-exclusive deals and flash sales. Through Mobile App Scraping Services, businesses can collect this app-specific data and integrate it into pricing models for more competitive offerings.
Tools Used in Scraping Alcohol Data
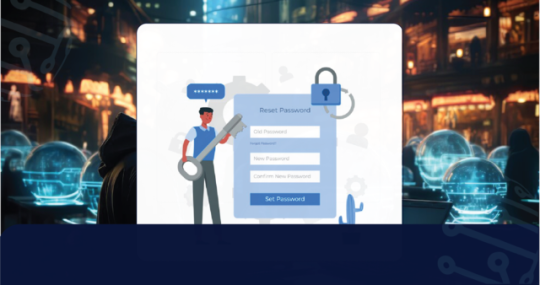
As the global wine and liquor industry shifts toward data-driven strategies, businesses increasingly rely on robust tools specifically designed for Web Scraping Liquor or Alcohol Data and compiling Liquor or Alcohol Price Review Datasets. These tools must be capable of handling dynamic content, JavaScript-rendered pricing modules, and anti-bot mechanisms such as CAPTCHA and IP restrictions.
Modern Web Scraping Services employ scalable and flexible infrastructures to crawl thousands of wine and liquor product pages daily. Whether it's eCommerce platforms, regional distributor websites, or global wine trading portals, these tools ensure high data accuracy and consistency.
To Scrape wine sales data 2025 effectively, companies integrate Web Scraping API Services to fetch real-time pricing, availability, and promotional information. APIs ensure continuous, structured delivery of data, enabling wine importers and marketers to Analyze wine sales trends 2025 and react quickly to shifting market conditions.
In addition, Mobile App Scraping Services are used to extract pricing and offer-related content from liquor and wine delivery apps—many of which feature app-exclusive rates or localized offers not visible on web portals.
Advanced Wine industry data scraper tools not only collect data but also normalize and clean it, enabling organizations to Extract wine marketing data efficiently for analytics dashboards, CRM systems, or pricing intelligence platforms. These tools help businesses Scrape Alcohol Price Data Intelligence from multi-language and multi-currency platforms with high accuracy.
By leveraging the power of Web scraping wine pricing trends, companies in the liquor and wine space gain a competitive edge—ensuring they stay ahead of pricing shifts, seasonal fluctuations, and consumer demand in every region.
ArcTechnolabs integrates these modern tools with precision to deliver actionable insights from global alcohol data sources.
Why Choose ArcTechnolabs?
At ArcTechnolabs, we offer end-to-end Web Scraping Services tailored specifically to the wine and alcohol industry. Our expertise includes building powerful Wine industry data scraper tools designed for both global and regional coverage. We provide robust Web Scraping API Services that automate real-time data delivery, ensuring you have access to the most up-to-date information at all times. Our Mobile App Scraping Services are fully scalable, enabling you to access exclusive pricing and promotional data often locked behind mobile platforms.
We also utilize advanced scraping techniques to extract data from JavaScript-heavy websites and protected sources, overcoming typical scraping challenges like CAPTCHAs and geo-restrictions. For businesses looking to make informed decisions, we deliver custom dashboards and analytics tools that help you Analyze wine sales trends 2025 effortlessly.'
Whether you want to Scrape BookMyShow movie ticket data today or Scrape Alcohol Price Data Intelligence tomorrow, ArcTechnolabs builds flexible, industry-specific scraping solutions that evolve with your business goals.
Conclusion
The global wine market is rich in opportunity—but only for those who stay informed. By leveraging Web scraping wine pricing trends, importers and sommeliers gain the competitive advantage needed to forecast accurately, price competitively, and market intelligently. With ArcTechnolabs as your data partner, you can transform fragmented online wine listings into actionable intelligence that fuels your business success. Ready to unlock real-time wine pricing insights? Contact ArcTechnolabs today for a custom scraping solution built for your market!
Source >> https://www.arctechnolabs.com/wine-pricing-data-scraping-global-trends.php
🚀 Fuel your growth with real-time data! 📩 [email protected] | 📞 +1 424 377 7584 ArcTechnolabs – Data that drives decisions.
#WebScrapingWinePricingTrends#ScrapeAlcoholPriceDataIntelligence#LiquorOrAlcoholPriceReviewDatasets#ExtractWineMarketingData#WebScrapingLiquorOrAlcoholData#WebScrapingAPIServices#ArcTechnolabs
0 notes
Text

📱 Gain Competitive Advantage with Mobile App Scraping at Scale
With over 5 million apps on app stores and billions of user interactions daily, mobile app data holds the key to next-gen insights for growth, product strategy, and customer behavior analysis.
🔍 Key capabilities of Mobile App Scraping:
🚀 Extract real-time reviews, ratings & user sentiment 📦 Monitor pricing, features, downloads, and updates 🧠 Enable AI-driven user behavior & competitor analysis 🌐 Track app trends across iOS, Android, and third-party platforms 💡 Power your BI dashboards with mobile app intelligence
💬 Whether you're in eCommerce, food delivery, travel, or fintech—mobile app data is your gateway to actionable intelligence
0 notes
Text
AI & Machine Learning | Quick Commerce Data Scraping Role
Introduction
The rise of Quick Commerce (Q-Commerce) has transformed the way businesses manage inventory, pricing, and customer demands. With ultra-fast delivery expectations from platforms like Blinkit, Instacart, Getir, Swiggy Instamart, and Gorillas, businesses need real-time, data-driven insights to stay ahead. AI (Artificial Intelligence) and ML (Machine Learning) are revolutionizing data scraping for quick commerce by automating processes, enhancing accuracy, and providing predictive insights.
Retail Scrape, a leader in AI-driven web and mobile app scraping, enables businesses to extract valuable data to optimize pricing, monitor stock availability, and analyze market trends. This blog explores how AI & ML are reshaping Quick Commerce Data Scraping to drive smarter business decisions.
Why AI & ML are Crucial for Quick Commerce Scraping
Automating Data Extraction
Handling CAPTCHA & Anti-Bot Protections
Predicting Pricing & Demand Trends
Processing Large-Scale Data Efficiently
Ensuring High Data Accuracy
How AI & ML Enhance Quick Commerce Data Scraping
1. AI-Driven Dynamic Pricing Scraping
2. Machine Learning for Inventory Tracking
3. AI-Powered Competitor Analysis
4. ML-Driven Sentiment Analysis for Customer Insights
Challenges in AI-Powered Quick Commerce Scraping & Solutions
AI-powered solutions help overcome key web scraping challenges. When websites change structure often, machine learning scrapers adapt automatically. For tough anti-scraping tools like CAPTCHAs and bot detection, AI uses rotating proxies and human-like interactions. Real-time pricing and stock changes are tracked using scheduled scrapers.
Geo-blocked deals and personalized offers are accessed with geo-targeted proxies and behavior replication. Large data volumes and API limits are handled with request throttling and cloud-based AI scraping. AI also cleans messy data using NLP to turn raw HTML into insights. Finally, data from web, mobile, and APIs is unified using AI tools that merge different formats like JSON and CSV.
Industries Benefiting from AI & ML in Quick Commerce Scraping
1. eCommerce & Retail
2. FMCG & Grocery Brands
3. Market Research & Analytics Firms
4. Logistics & Supply Chain Companies
How Retail Scrape Leverages AI & ML for Data Scraping
Retail Scrape provides AI-driven Quick Commerce Data Scraping Solutions to help businesses harness actionable insights. Our offerings include:
1. Automated Web & Mobile App Scraping for Quick Commerce Data.
2. Competitor Price & Inventory Tracking with AI-Powered Analysis.
3. Real-Time Data Extraction & API Integration.
4. Custom Dashboards for Data Visualization & Predictive Insights.
Conclusion
AI and ML are transforming Quick Commerce Data Scraping by enabling businesses to make smarter, data-driven decisions. With the rapid evolution of Blinkit, Getir, Instacart, and Swiggy Instamart, leveraging AI-powered scraping ensures businesses stay ahead of competitors.
With Retail Scrape’s AI-driven solutions, companies can efficiently extract, analyze, and utilize real-time pricing, inventory, and market insights for growth and profitability.
Looking to integrate AI-powered Quick Commerce Scraping? Contact Retail Scrape today for cutting-edge data extraction solutions!
Read more >>https://www.retailscrape.com/ai-machine-learning-quick-commerce-data-scraping.php
officially published by https://www.retailscrape.com/.
#QuickCommerceDataScrapingSolutions#AutomatingDataExtraction#DynamicPricingScraping#ScrapeWithAIAndML#MobileAppScraping#QuickCommerceData#RevolutionizingDataScraping#RealTimeDataExtraction#BlinkitDataExtraction#InstacartDataExtraction#SwiggyInstamartDataExtraction
0 notes
Text
Scrape Flipkart Big Billion Days vs Amazon Great Indian Festival

Scrape Flipkart Big Billion Days Deals vs Amazon Great Indian Festival Offers
As the festive season in India approaches, the spotlight shines on two of the most significant online shopping events: Flipkart's Big Billion Days and Amazon's Great Indian Festival. Scrape Flipkart Big Billion Days Deals vs. Amazon Great Indian Festival Offers to know how these sales promise to deliver exceptional deals across various categories, captivating millions of shoppers eager to seize the best bargains.
In 2024, both platforms are expected to see significant traffic, with Flipkart anticipated to attract around 100 million visitors during its sale, while Amazon aims for approximately 85 million. Extract Flipkart Big Billion Days Deals and offer to get this year's events highlighted on various offerings, from electronics to fashion, as consumers gear up for festive shopping.
Market trends obtained through Ecommerce Data Scraping Service indicate a growing preference for online shopping, especially among younger demographics, with mobile commerce leading the way. Data insights reveal Flipkart typically sees higher engagement in smaller cities, while Amazon appeals strongly to urban customers.
The article will include charts and graphs illustrating visitor numbers, popular product categories, and purchasing patterns to help visualize these trends. This comparison will guide consumers in making informed decisions and showcase the competitive landscape between these two e- commerce giants in India.
Our Analysis Shows
Visitor Traffic: Flipkart is expected to attract around 100 million visitors, while Amazon targets about 85 million, showcasing Flipkart's market strength.
Product Categories: Both platforms offer discounts in electronics and fashion, with Flipkart providing more bottomless deals on electronics and Amazon focusing on fashion and lifestyle.
Consumer Preferences: Flipkart engages more shoppers in tier-2 and tier-3 cities, while Amazon appeals to urban customers, reflecting different target demographics.
Market Trends: Mobile commerce is rising, with more shoppers using apps, emphasizing the need for user-friendly mobile interfaces.
Promotional Strategies: Flipkart relies on flash sales, while Amazon offers a broader range of deals throughout the festival, catering to various shopping habits.
Overview of Sales Events in 2024
Flipkart Big Billion Days: Since its inception in 2014, Flipkart's Big Billion Days has established itself as one of India's most significant sales events. Scheduled from September 27 to October 06, 2024, this sale features flash deals, early access for select customers, exclusive brand launches, and discounts on various products.
Amazon Great Indian Festival: From September 27 to October 09, 2024, Amazon's festival has rapidly gained traction. It attracts customers with substantial discounts, Prime member benefits, and an extensive range of product categories, making it a formidable competitor to Flipkart.
Sales Duration and Timing
Sales EventDurationStart DateFlipkart Big Billion DaysSeptember 27 - October 06, 2024September 27, 2024Amazon Great Indian FestivalSeptember 27 - October 09, 2024September 27, 2024
Both sales events span about a week, providing ample time for consumers to explore deals across various product categories.
Category-wise Discounts and Offers
CategoryFlipkart Big Billion Days (Average Discount)Amazon Great Indian Festival (Average Discount)Electronics30% - 55%25% - 50%Fashion40% - 70%30% - 60%Home Appliances30% - 60%25% - 50%Groceries10% - 25%15% - 30%Beauty & Personal Care25% - 40%15% - 35%Books35% - 55%30% - 45%
Essential Offers and Deals for 2024
Flipkart: Shoppers can expect significant discounts of up to 55% on smartphones from leading brands such as Samsung, Apple, and Xiaomi. Flagship models like the iPhone series and Galaxy devices are prominently featured, attracting tech-savvy customers. In addition to these discounts, Flipkart offers bank promotions that provide further savings on select purchases, making it a prime destination for electronic goods.
Amazon: Scrape Amazon Great Indian Festival Promo Codes to know how this platform will feature discounts of up to 50% on laptops, tablets, and various accessories. The sale includes popular brands like Dell, HP, and Lenovo, catering to a diverse audience of tech enthusiasts. Web Scraping Amazon Product data shows attractive exchange offers and no-cost EMI options, allowing customers to upgrade their devices affordably and conveniently.
Flipkart: Extracting Flipkart Product data reveals that the fashion segment boasts substantial discounts of up to 70% on clothing, footwear, and accessories from popular brands like Adidas, Levi's, and Allen Solly. Flipkart is expected to launch exclusive collections and special offers on bundled purchases, encouraging customers to shop more and take advantage of the savings.
Amazon: In line with Flipkart, Amazon will present exciting discounts of up to 60% across a wide range of fashion items. Additionally, cashback offers for Prime members enhance the shopping experience. Data from Ecommerce Product Datasets showed that the category encompasses various styles, including ethnic wear, western apparel, and accessories, appealing to a broad audience.
Flipkart: Customers can expect 30% to 60% discounts on essential home appliances like refrigerators, washing machines, and kitchen gadgets. Flipkart offers financing options on higher-value items to facilitate upgrades, making it easier for consumers to invest in new appliances without straining their budgets.
Amazon: Competing closely with Flipkart, Amazon will provide discounts of up to 50% on home appliances as per Price Monitoring Service. Customers can use additional exchange schemes to trade in their old appliances for better deals, making it a smart choice for those looking to upgrade.
Flipkart: The grocery segment will see discounts ranging from 10% to 25% on everyday essentials. Exclusive bundles will be available during the sale, catering to consumers looking for popular pantry staples and healthy foods, enhancing their shopping experience.
Amazon: Customers can expect competitive pricing and bundled offers on grocery items, with discounts between 15% and 30%. Amazon emphasizes its Prime Pantry service, providing special offers and discounts for Prime members, making shopping for groceries easier.
Flipkart: This category features discounts between 25% and 40% on skincare and cosmetics products from popular brands like L'Oréal, Maybelline, and M.A.C. Exclusive beauty boxes that bundle popular products are expected to attract buyers, offering both variety and savings.
Amazon: In this segment, Amazon offers attractive deals on beauty products, with discounts ranging from 15% to 35%. This includes exclusive brand launches and limited-time offers, which create excitement among beauty enthusiasts.
Flipkart: The book category will see discounts of 35% to 55%, appealing to avid readers with a vast selection ranging from bestsellers to educational materials. The focus on diverse genres and formats, including print and e-books, enhances the appeal for various reader demographics.
Amazon: Like Flipkart, Amazon offers discounts of 30% to 45% on books, including exclusive deals on Kindle devices and e-books. This makes Amazon a favorite among book lovers, especially those who prefer digital reading formats.
This comprehensive overview illustrates how Flipkart and Amazon cater to different consumer needs across various categories, offering competitive pricing and unique deals during their festive sales.
Consumer Preferences and Market Trends in 2024
Consumer Preference
Recent surveys reveal significant insights into consumer preferences between Flipkart and Amazon, particularly during festive sales events.
Flipkart's Strengths:
Electronics & Fashion: Flipkart is increasingly considered the go-to platform for purchasing electronics and fashion items. This preference stems from various factors, including competitive pricing, attractive discounts, and exclusive brand partnerships. Consumers often report finding better deals on flagship electronics and trendy fashion items on Flipkart, especially during significant sales like the Big Billion Days.
Survey Insights: According to the data, approximately 60% of respondents preferred shopping on Flipkart during sales. This statistic highlights Flipkart's strong brand loyalty among Indian consumers, particularly in categories like smartphones and clothing.
Amazon's Advantages:
Vast Product Selection: Amazon is favored for its extensive catalog, offering various items beyond electronics and fashion. Customers appreciate the convenience of finding almost anything they need, from household goods to niche products, all in one place.
Customer Service: Web Scraping Amazon Great Indian Festival Shopping Offers reveals that Amazon's reputation for excellent customer service, including easy returns and reliable delivery, also significantly attracts consumers. According to the survey, about 40% of respondents preferred Amazon, which underscores its strong appeal in the market.
Market Trends
The e-commerce landscape in India is rapidly evolving, particularly during the festive season, which presents a lucrative opportunity for both Flipkart and Amazon.
Projected Growth: The overall e-commerce market in India is projected to reach $99 billion by 2024. This growth is driven by increasing internet penetration, the proliferation of smartphones, and changing consumer shopping behaviors. The festive season significantly contributes to this growth, as many consumers shop online for gifts, clothing, electronics, and household items.
Festive Sales Impact: According to Statista, online sales during the festive season of 2024 are estimated to reach $10 billion. This figure reflects the growing trend of consumers turning to online platforms for shopping during significant festivals. Flipkart and Amazon are expected to dominate this space, collectively accounting for approximately 70% of this revenue.
Competition and Innovation: To capture a larger share of the festive sales, both companies invest heavily in marketing, promotions, and logistics. They also strive to enhance the shopping experience through features like personalized recommendations, flash sales, and exclusive brand launches.
In summary, while consumer preferences show a distinct inclination toward Flipkart for electronics and fashion, Amazon's broad product range and superior customer service continue to attract a loyal customer base. Both platforms are poised to play pivotal roles in the booming e-commerce market, particularly during the festive season, reflecting the dynamic nature of online shopping in India.
Comparison of Visitor Traffic During Flipkart Big Billion Days and Amazon Great Indian Festival
Here's a 2024 comparison of visitor traffic during Flipkart's Big Billion Days and Amazon's Great Indian Festival, focusing on key metrics and insights:
2024 Comparison of Visitor Traffic
E-commerce PlatformEstimated Visitors (2024)Percentage Increase from 2023Peak Traffic TimeTarget AudienceFlipkart100 million15%Day 1 of the saleTier 2 and Tier 3 cities, price-sensitive shoppersAmazon85 million12%Mid-sale peak daysUrban customers, diverse demographics
Detailed Insights
1. Estimated Visitors:
Flipkart: Expected to attract around 100 million visitors, reflecting its robust engagement strategies tailored to Indian consumers, particularly in smaller cities.
Amazon: Anticipating 85 million visitors, focusing on urban areas, emphasizing convenience and a diverse range of products.
2. Percentage Increase:
Flipkart: Anticipated 15% increase in traffic from 2023, driven by heavy marketing campaigns and attractive discounts across multiple categories.
Amazon: A 12% increase in visitor traffic, showcasing its enduring appeal, particularly among Prime members, who benefit from exclusive offers.
3. Peak Traffic Time:
Flipkart: Traffic will likely peak on the first day of the sale as shoppers rush to take advantage of limited-time deals, particularly on electronics and fashion.
Amazon: Significant traffic is expected during mid-sale peak days, particularly for flash sales and promotional events to boost engagement.
4. Target Audience:
Flipkart: Primarily attracts consumers from Tier 2 and Tier 3 cities, where online shopping is gaining popularity. The platform's focus on value-for-money deals resonates well with price-sensitive shoppers.
Amazon: It appeals predominantly to urban customers, a diverse demographic that appreciates a broad selection of products, including premium brands and exclusive launches.
Data Insights and Statistics
Sales Growth: In 2024, Flipkart aims to sell over 18 million smartphones during its Big Billion Days, marking a 20% increase from the previous year due to expanded product offerings and attractive discounts. Meanwhile, Amazon expects a rise in Prime memberships as more consumers take advantage of exclusive deals and benefits during the Great Indian Festival. This trend reflects India's growing demand for online shopping, promising solid sales growth for both platforms.
Cart Abandonment Rates: A recent study showed that cart abandonment rates during festive sales remain a challenge for both platforms, with rates hovering around 65%. However, both platforms are implementing strategies to reduce this through personalized discounts and notifications.
Conclusion
The Flipkart Big Billion Days and Amazon Great Indian Festival in 2024 promise to deliver thrilling shopping experiences for consumers, with substantial discounts across various categories. While Flipkart is poised to dominate in electronics and fashion, Amazon continues to attract customers with its comprehensive range of products and exceptional delivery services. As both platforms evolve and adapt to consumer needs, they will further enrich the online shopping landscape, ensuring that customers enjoy the best deals during the festive season.
Experience top-notch web scraping service and mobile app scraping solutions with iWeb Data Scraping. Our skilled team excels in extracting various data sets, including retail store locations and beyond. Connect with us today to learn how our customized services can address your unique project needs, delivering the highest efficiency and dependability for all your data requirements.
Source: https://www.iwebdatascraping.com/scrape-flipkart-big-billion-days-vs-amazon-great-indian-festival.php
#ScrapeFlipkartBigBillionDaysDeals#AmazonGreatIndianFestivalOffers#ExtractFlipkartBigBillionDaysDealsAndOffers#ScrapeAmazonGreatIndianFestivalPromoCodes#WebScrapingAmazonProductData#ExtractingFlipkartProductData#EcommerceDataScrapingService
0 notes
Text
Web Scraping 102: Scraping Product Details from Amazon
Now that we understand the basics of web scraping, let's proceed with a practical guide. We'll walk through each step to extract data from an online ecommerce platform and save it in either Excel or CSV format. Since manually copying information online can be tedious, in this guide we'll focus on scraping product details from Amazon. This hands-on experience will deepen our understanding of web scraping in practical terms.
Before we start, make sure you have Python installed in your system, you can do that from this link: python.org. The process is very simple just install it like you would install any other application.
Install Anaconda using this link: https://www.anaconda.com/download . Be sure to follow the default settings during installation. For more guidance, please click here.
We can use various IDEs, but to keep it beginner-friendly, let's start with Jupyter Notebook in Anaconda. You can watch the video linked above to understand and get familiar with the software.
Now that everything is set let’s proceed:
Open up the Anaconda software and you will find `jupyter notebook` option over there, just click and launch it or search on windows > jupyter and open it.
Steps for Scraping Amazon Product Detail's:
At first we will create and save our 'Notebook' by selecting kernel as 'python 3' if prompted, then we'll rename it to 'AmazonProductDetails' following below steps:
So, the first thing we will do is to import required python libraries using below commands and then press Shift + Enter to run the code every time:
Let's connect to URL from which we want to extract the data and then define Headers to avoid getting our IP blocked.
Note : You can search `my user agent` on google to get your user agent details and replace it in below “User-agent”: “here goes your useragent line” below in headers.
Now that our URL is defined let's use the imported libraries and pull some data.
Now, let's start with scraping product title and price for that we need to use `inspect element` on the product URL page to find the ID associated to the element:
The data that we got is quite ugly as it has whitespaces and price are repeated let's trim the white space and just slice prices:
Let's create a timespan to keep note on when the data was extracted.
We need to save this data that we extracted, to a .csv or excel file. the 'w' below is use to write the data
Now you could see the file has been created at the location where the Anaconda app has been installed, in my case I had installed at path :"C:\Users\juver" and so the file is saved at path: "C:\Users\juver\AmazonProductDetailDataset"
Instead of opening it by each time looking for path, let's read it in our notebook itself.
This way we could extract the data we need and save it for ourselves, by the time I was learning this basics, I came across this amazing post by Tejashwi Prasad on the same topic which I would highly recommend to go through.
Next, we’ll elevate our skills and dive into more challenging scraping projects soon.
0 notes
Text
Empower the Surge of Online Alcohol Retail in the UK by Web Scraping Liquor Data
The Alcohol eCommerce sector has experienced rapid expansion, a trend accentuated by the pandemic. Post-pandemic, factors like convenience, safety, and home delivery gained prominence, propelling alcohol sales through eCommerce. In the UK, Kantar noted a £261 million surge in rising sales, with online and convenience stores emerging as the key beneficiaries. Intriguingly, the IWSR Drinks Market Analysis Report 2022 highlighted a global preference for websites over apps when purchasing alcohol online, except in China and Brazil. Notably, in the UK, major online alcohol purchases occur via retailer websites, not apps.
Research Approach
Our research was conducted through liqour data scraping, spanning February 2022 to June 2022. The focus of our study encompassed two prominent grocery retailers, namely Tesco and Ocado, along with three noteworthy grocery apps, namely Gorillas, Weezy, and Getir. The specific category under scrutiny throughout our analysis was alcohol.
Identifying The Alcohol Price Leader
Tesco's Leadership In Alcohol Pricing
Throughout February to June 2022, Tesco consistently established itself as the front-runner in price leadership within the Alcohol category. Leveraging e-commerce data scraping, Tesco secured the lowest prices for 38.9% of its products. Ocado followed closely with 33.8%, while Gorillas exhibited the most minor dominance, leading in price for only 5.6% of its alcohol offerings.
Nonetheless, Tesco's grip on price leadership experienced a gradual erosion over the months. Commencing in February,e-commerce data scraping revealed Tesco's hold on the lowest price for 44% of its products, which tapered to just over 36% by June. Conversely, Ocado depicted an inverse trajectory—starting at a 32% price leadership in February, climbing to 35.3% by June.
An intriguing contender, illuminated through e-commerce data scraping, was Getir. Commencing with a modest 8.2% price leadership for its products in February, Getir's proportion progressively expanded, culminating at 14.5% in June.
Retailers Employing Discounts To Bolster Alcohol Sales
Leveraging discounts using liquor product data collection proves to be an effective strategy to attract consumers impacted by inflation. Through loyalty card discounts, reward vouchers, and various promotional tactics, retailers enhance the allure of their products, rendering them more competitive and appealing to customers. Maintaining competitiveness necessitates retailers' awareness of their rivals' discount offerings. Moreover, price monitoring helps comprehend the potential ramifications of substantial discounting and its influence on profit margins.
A wave of European and UK startups, including Jiffy, Dija, Weezy, Zapp, Getir, and Gorillas, entered the scene with a shared commitment: to provide the swiftest and most cost-effective grocery delivery services.
Our dataset uncovered intriguing discounting dynamics among these players. Gorillas maintained a discounting strategy in line with its competitors. Conversely, Getir appeared to adopt a more aggressive approach, veering towards deep discounting. Notably, Getir consistently offered the highest discounts throughout the observed period. In April, their discounts surged to nearly 9% more than those of Ocado—the runner-up in discounting.
As previously discussed, the period from February to June saw Getir securing price leadership. It's plausible that their strategy of deep discounting contributed to this accomplishment. In contrast, Gorillas opted for a different route, showcasing the lowest and almost negligible discounting practices.
Observing Price Index Trends Over Five Monthss
Our focus shifted towards analyzing Price Index (PI) trends among these five retailers, encompassing February to June 2022. It enabled us to gauge the fluctuations in alcohol prices over this period.
Please note: Retailers operating at the 100% mark indicated they were selling at an optimal price, refraining from undercutting the market. The pricing sweet spot rested between 95% and 105%. Deviating lower would potentially jeopardize profit margins while exceeding this range indicated that the retailer needed to position more competitively.
Price Index Insights: Retailers' Strategic Positioning
Among the retailers, Weezy displayed the most optimal Price Index, residing within the 100% to 102% range.
Conversely, Gorillas held the lowest Price Index, from 89% to 91%.
Getir, initially boasting a lower Price Index of 96.1% in February, progressively climbed to surpass 110% in April, May, and June.
Agility In Price Adjustments: Identifying Leaders
Stability In Pricing Trends Among Retailers
Predominantly, most retailers adhered to a consistent pricing trajectory using liquor product data scraping, maintaining a degree of competitive alignment. Their pricing approaches were relatively well-matched.
Yet, Gorillas stood out for implementing significant price adjustments in specific months. Notably, they enacted a notable reduction of 3.8% in prices in March. Subsequently, in May, Gorillas increased 5.5% in their pricing.
In that same eventful May, Weezy embarked on a distinctive strategy, considerably slashing prices by 10%. This move widened the gap between Gorillas and Weezy.
Preserving Sales Through Stock Availability Management
Diverse Stock Availability Among Retailers
Our data analysis unveiled distinct levels of stock availability across the retailers. Ocado, in particular, maintained the highest availability throughout the observed five months. At a robust 100%, their stock levels gradually receded, concluding at 95.8% by June.
Tesco, on the other hand, encountered a pronounced decline in availability during May and June. At 97%, availability dwindled to 92-93%.
Gorillas consistently exhibited the lowest stock availability, fluctuating between 90% and 94% across the months.
Weezy demonstrated steadfast consistency, upholding a consistent 95% availability throughout five months.
Concluding Insights
In the UK market, a favorable inclination towards online alcohol purchases is evident, predominantly catalyzed by shifts in consumer behavior prompted by the pandemic. According to the IWSR Drinks Market Analysis Report 2022, markets primarily driven by websites, like the UK, prioritize a wide product range and competitive pricing. Both these factors wield significant influence in purchase decisions. Conversely, consumers in app-centric markets hold distinct preferences. While price remains a consideration, it is less vital than convenience and speed.
Product Data Scrape is committed to upholding the utmost standards of ethical conduct across our Competitor Price Monitoring Services and Mobile App Data Scraping operations. With a global presence across multiple offices, we meet our customers' diverse needs with excellence and integrity.
#WebScrapingLiquorData#ScrapeUKAlcoholRetailIndustry#WebScrapingRetailWebsitesData#ScrapeUKRetailTrends#UKLiquorDataScraper#ScrapeLiquorData
0 notes
Text
Amazon Data Scraping: Understanding the Process, Advantages, and Challenges
Amazon Data Scraping: Understanding the Process, Advantages, and Challenges
Dec 01, 2023
Introduction
In the global e-commerce giant Amazon, data scraping has emerged as a strategic tool for extracting invaluable insights. This process involves automated extraction from the platform, providing businesses with a comprehensive understanding of market trends, consumer behavior, and product dynamics. eCommerce App Data Scraping Services facilitate nuanced market research, competitive pricing intelligence, and vigilant product catalog monitoring. Despite its advantages, challenges include legal considerations, dynamic website structures, and anti-scraping measures. Navigating this landscape demands a balanced approach, leveraging the benefits while adhering to ethical practices and legal compliance
Decoding The Mechanics Of Amazon Data Scraping: Insights Into Operation And Impact
Amazon data scraping operates as a systematic process designed to extract critical information from the vast expanse of the e-commerce giant's platform. The intricacies of how this mechanism functions lie in automated scripts that navigate through Amazon's web pages, systematically collecting data on products, pricing, customer reviews, and more. These scripts mimic human interaction but do so at scale and speed, allowing for retrieving a wealth of data in real-time. By tapping into Amazon's product listings and customer interactions, businesses can gain valuable insights into market trends, consumer preferences, and competitive landscapes. However, the effectiveness of Amazon data scraping is contingent on adeptly handling challenges such as evolving website structures, legal considerations, and anti-scraping measures implemented by Amazon to safeguard its data. In navigating this landscape, businesses can responsibly harness the power of data scraping, maximizing its potential for strategic decision-making and market positioning.
Navigating Hurdles And Remedies In Extracting Data From Amazon
Scraping Amazon, or any website, can present several challenges due to legal, ethical, and technical considerations. Amazon's terms of service prohibit web scraping, and engaging in scraping activities may lead to legal consequences. However, for educational purposes, it's essential to understand the challenges and potential solutions:
Challenges:
Legal and Ethical Issues:
Terms of Service (ToS): Amazon explicitly prohibits web scraping in its terms of service. Violating these terms can lead to legal action against the scraper.
Intellectual Property Concerns: Scraping product information may involve collecting copyrighted or proprietary data.
Anti-Scraping Mechanisms:
Bot Detection: Amazon employs anti-scraping mechanisms, including bot detection tools, to identify and block automated scraping activities.
IP Blocking: Amazon may block IP addresses engaging in scraping to prevent unauthorized access.
Dynamic Content and AJAX:
Dynamic Loading: Amazon's website uses dynamic content loading through AJAX, making it challenging to scrape data using traditional methods.
CAPTCHA Challenges:
CAPTCHA Protection: Frequent scraping attempts may trigger CAPTCHA challenges, making automated data collection more complex.
Data Volume and Rate Limiting:
Rate Limiting: Amazon may implement rate limiting to control the number of requests from a single IP address, hindering large-scale scraping.
Solutions:
Respect Terms of Service:
Ethical Scraping: Adhere to the website terms of service you are scraping. Avoid activities that violate these terms to maintain legal and ethical integrity.
Use APIs if Available:
API Integration: If Amazon provides an API for data access, consider using it instead of scraping. APIs are designed for data retrieval and are more reliable and legal.
Proxy Rotation:
Proxy Servers: Rotate IP addresses using proxy servers to avoid detection and IP blocking. This helps distribute requests across multiple IPs, reducing the likelihood of being flagged.
CAPTCHA Solving:
CAPTCHA Solvers: Implement CAPTCHA-solving mechanisms to overcome challenges. Be cautious, as solving CAPTCHAs programmatically may violate terms of service.
Rate Limiting and Throttling:
Politeness and Throttling: Implement polite scraping by adhering to rate limits and introducing delays between requests to avoid triggering anti-scraping measures.
Dynamic Content Handling:
Headless Browsing: Use headless browsers or browser automation tools like Selenium to handle dynamic content loading and AJAX requests.
Legal Consultation:
Legal Advice: Before engaging in scraping activities, seek legal advice to understand the potential legal implications and risks.
Always prioritize ethical and legal considerations when scraping websites, and be aware that terms of service can change. It's crucial to stay informed and adjust your practices accordingly.
Advantages Gained From Extracting Insights Through Amazon Data
Using eCommerce App Data Scraping Services to scrape Amazon data can offer several benefits, providing valuable insights for various purposes. Here are detailed explanations of the benefits:
Market Research:
Product Trends: Analyzing Amazon data helps identify trending products, allowing businesses to stay informed about consumer preferences and market demands.
Competitor Analysis: Scrutinizing competitors' product listings, pricing strategies, and customer reviews provides a comprehensive understanding of the competitive landscape.
Price Monitoring and Optimization:
Dynamic Pricing: Tracking product prices on Amazon allows businesses to adjust their pricing strategies dynamically, staying competitive and maximizing profitability.
Promotional Insights: Monitoring discounts, promotions, and pricing fluctuations helps businesses strategize their promotional activities.
Inventory Management:
Stock Levels: Scraping data from Amazon enables businesses to monitor the availability of products, helping optimize inventory levels and prevent stockouts or overstock situations.
Seasonal Demand: Understanding seasonal trends through historical data assists in planning inventory for peak periods.
Customer Reviews and Sentiment Analysis:
Product Feedback: Analyzing customer reviews provides insights into product satisfaction, joint issues, and feature preferences, helping businesses enhance product quality and customer experience.
Brand Reputation: Monitoring overall sentiment towards brands on Amazon aids in managing and improving brand reputation.
Marketing and Advertising Strategies:
Keyword Optimization: Extracting data helps identify high-performing keywords used by competitors, contributing to optimizing product listings and advertisements.
Ad Spend Analysis: Analyzing Amazon advertising data enables businesses to evaluate the effectiveness of their ad campaigns and refine their advertising strategies.
New Product Development:
Gap Analysis: Examining gaps or unmet needs in the market through Amazon data allows businesses to identify opportunities for developing new products or improving existing ones.
Feature Preferences: Understanding customer preferences from reviews aids in designing products that align with consumer expectations.
E-commerce Analytics:
Sales Performance: Tracking sales data on Amazon provides valuable insights into the performance of products, helping businesses assess the success of their offerings.
Geographic Analysis: Understanding regional preferences and sales patterns contributes to effective geographic targeting and expansion strategies.
Strategic Decision-Making:
Data-Driven Decision-Making: Amazon data serves as a foundation for making informed business decisions and guiding strategies related to pricing, inventory, marketing, and overall business operations.
Risk Mitigation: Identifying potential challenges and market shifts early through data analysis allows businesses to mitigate risks proactively.
While these benefits are significant, it's crucial to approach eCommerce App Data Scraping Services with ethical considerations and compliance with terms of service to ensure responsible and lawful data extraction.
Employing Web Scraping For The Extraction Of Amazon Data
Utilizing eCommerce App Data Scraping Services for collecting data from Amazon offers a range of advantages, enabling users to gather valuable information for various purposes. Here's a detailed exploration of the benefits and applications of using web scraping for Amazon data collection:
Product Research and Analysis:
Comprehensive Product Data: Web scraping allows for extracting detailed product information, including specifications, features, and customer reviews, aiding in thorough product research.
Market Trends: Analyzing scraped data enables users to identify emerging market trends, popular products, and consumer preferences.
Competitor Intelligence:
Price Monitoring: Web scraping facilitates real-time tracking of competitor prices, helping businesses stay competitive by adjusting their pricing strategies accordingly.
Assessing Strategies: By examining competitor product listings, promotions, and customer reviews, businesses can gain insights into their competitors' strategies and market positioning.
Inventory Management:
Stock Availability: Scraping Amazon data allows for monitoring stock levels, aiding in effective inventory management, and preventing stockouts or excess inventory.
Seasonal Demand Prediction: Historical data can be analyzed to predict seasonal fluctuations in demand, assisting in optimizing inventory for different periods.
Dynamic Pricing Strategies:
Adaptive Pricing: With real-time price data, businesses can implement dynamic pricing strategies, adjusting prices based on market conditions and competitor activities.
Profit Maximization: Web scraping enables businesses to identify optimal price points that balance competitiveness and profitability.
Customer Insights and Reviews:
Feedback Analysis: Extracting customer reviews provides valuable insights into product satisfaction, joint issues, and areas for improvement.
Customer Sentiment: Analyzing sentiment in reviews helps businesses understand how customers perceive products and make data-driven decisions to enhance customer satisfaction.
Marketing Optimization:
Keyword Analysis: Web scraping assists in identifying high-performing keywords used in product listings and customer reviews, contributing to the optimization of marketing content.
Ad Campaign Evaluation: Scrapped data from Amazon advertising can be analyzed to assess the effectiveness of marketing campaigns and refine strategies.
Data-Driven Decision Making:
Strategic Planning: Businesses can make informed decisions by relying on scraped data for market analysis, identifying opportunities, and mitigating potential risks.
Operational Efficiency: Automating data collection through web scraping streamlines decision-making and enhances operational efficiency.
E-commerce Analytics:
Sales Performance: Scrapped data provides insights into the performance of products, helping businesses evaluate the success of their offerings on Amazon.
Geographic Trends: Regional data analysis aids in understanding geographic sales patterns, facilitating targeted marketing efforts.
It's essential to approach eCommerce App Data Scraping Services responsibly, respecting the website's terms of service and adhering to ethical standards. Additionally, regular updates to the scraping process may be necessary to adapt to changes in the website structure or anti-scraping measures.
How Mobile App Scraping Can Help In Scraping Amazon Data?
Expertise in Web Scraping:
A specialized solution like Mobile App Scraping may have expertise in web scraping techniques, including handling dynamic content, bypassing anti-scraping mechanisms, and dealing with CAPTCHAs.
Customized Solutions:
Mobile App Scraping might offer customized scraping solutions tailored to client's specific requirements, ensuring that the extracted data meets their unique needs.
Data Accuracy and Quality:
A professional scraping service would prioritize data accuracy and quality, employing techniques to ensure the scraped information is reliable and up-to-date.
Compliance with Legal and Ethical Standards:
Mobile App Scraping, if reputable, would likely emphasize compliance with legal and ethical standards. This includes respecting the terms of service of websites like Amazon to avoid legal issues.
Scalability:
If Mobile App Scraping is a scalable service, it can handle large volumes of data scraping efficiently, making it suitable for businesses with diverse data needs.
Continuous Monitoring and Maintenance:
A reliable scraping solution would provide ongoing monitoring and maintenance to adapt to changes in the target website's structure or anti-scraping measures.
Data Analysis and Reporting:
Mobile App Scraping may offer additional services such as data analysis and reporting, providing clients with actionable insights from the scraped data.
Customer Support:
A reputable service would likely offer customer support to address issues, answer queries, and assist throughout the scraping process.
Before engaging with any data scraping service, it's essential to thoroughly research and ensure that the service adheres to legal and ethical standards. Additionally, verify the service's reputation, customer reviews, and any specific features or guarantees they provide. Always consider the terms of service of the websites you intend to scrape, including Amazon's policies on data extraction.
Conclusion
Mobile App Scraping emerges as a strategic partner for seamless Amazon data extraction. With expertise in navigating eCommerce App Data Scraping Services, a commitment to data accuracy, and a focus on legal compliance, Mobile App Scraping ensures tailored and scalable solutions. As businesses navigate the digital terrain, the significance of reliable, real-time insights cannot be overstated. Consider Mobile App Scraping as your trusted data scraping ally for a decisive edge. Empower your strategies, stay ahead of market trends, and make informed decisions. Contact Mobile App Scraping today for a personalized and impactful data extraction partnership that propels your business forward.
know more: https://www.mobileappscraping.com/amazon-data-scraping-process-the-advantages-and-challenges.php
#Amazondatascraping#AmazonDataScraper#ScrapeAmazonData#ExtractAmazonData#ExtractEcommerceData#AmazonDataCollection
1 note
·
View note
Text
Must-Have Scraping Tools for 2023: Unveiling the Top 6
The world of data is expanding at warp speed. By 2025, experts predict that the total amount of data in existence will skyrocket to over 180 zettabytes. To put that in perspective, in 2020, we were dealing with a mere 64.2 zettabytes. So, what's driving this data explosion? One word: the internet.
The Internet and the Data Boom
In 2020, the COVID-19 pandemic forced a massive shift in how we work and play. With the majority of the global population hunkering down at home, the internet became our lifeline, not just for work but also for entertainment. As a result, the generation and consumption of digital data reached unprecedented levels. And guess what? It's still on the rise. With so many people still working remotely, digital data is piling up faster than ever before.
Data Is King
Now, here's where it gets intriguing for businesses. With this explosion of data, companies can now dive deep into personal-level market behavior with pinpoint precision. As time goes on, data will only get bigger, and technology will get even more sophisticated. To stay ahead, businesses need to tap into this data goldmine. And one way to do that is through web scraping tools.
The Need for Web Scraping Tools
In this data-driven age, extracting data from the web is essential. There are countless ways to go about it, but web scraping tools are among the most powerful. They're your ticket to gathering data from multiple websites and mobile apps with incredible precision. Data is the lifeblood of any business strategy, and a web scraping tool can provide you with the vital data you need.
Choosing the Right Tool
Selecting the right scraping tool is crucial for your business's success. There are many options out there, but building your own tool is time-consuming and expensive. It's often more efficient to use an existing data scraping tool. To help you make the best choice, we've curated a list of the top 6 scraping tools you shouldn't miss.
Top 6 Data Scraping Tools
1. ApiScrapy
ApiScrapy is a trusted name in the world of data scraping. They offer a range of pre-built data scrapers for free, making it easy for businesses, researchers, and individuals to collect structured data in bulk. Whether it's eCommerce or social media, ApiScrapy provides tools to effortlessly gather data from various sources. Their AI-powered data scrapers are designed to handle diverse data needs, even from complex websites. ApiScrapy can handle it all, ensuring you get structured, ready-to-use data for analysis. Hundreds of web pages can be scraped within minutes, making it a top choice for data scraping.
2. Diffbot
Diffbot is an enterprise-level data scraping tool tailored for users with specific data crawling and screen scraping requirements. It excels at scraping websites with frequently changing HTML structures. Unlike other tools, Diffbot adapts to HTML changes, ensuring your web scrapers continue working smoothly. It visualizes webpages, making it resilient to structural changes as long as the page's appearance remains consistent. With support for APIs and AI extractors, Diffbot allows for accurate structured data extraction from any URL. It's a reliable choice for enterprise-level scraping used by companies like Microsoft and Adobe.
3. Dexi.io
Dexi.io is a visual web scraping software that uses computer vision instead of HTML parsing to identify relevant information on websites. It comes with built-in data flows and a web-based editor for configuring crawlers and extracting real-time data. Dexi.io is user-friendly, making it ideal for non-developer teams seeking rapid web data extraction and processing. This tool is known for its intuitive interface, making data extraction effortless. Marketers, data analysts, and HR personnel use Dexi.io to scrape web data in bulk.
4. Dataminer
Dataminer is a feature-rich browser extension that simplifies high-quality data extraction from multiple websites. With an intuitive user interface, Dataminer allows you to create over 60,000 data extraction rules with just a few clicks. You can effortlessly collect text from any web page, click on buttons and links automatically, navigate subpages, and scrape data from them. Dataminer is known for its ease of use and efficiency, making data scraping a breeze.
5. Mozenda
Mozenda is a web scraping tool designed for businesses needing data from various sources. It collaborates with 30% of Fortune 500 companies on large-scale projects such as pricing monitoring and market research. Mozenda helps businesses acquire the information necessary for data-driven decision-making, whether it's competitive pricing data or strategic insights. While it comes at a higher price, Mozenda offers robust features for organizing and preparing data files for publication. Its point-and-click user interface simplifies real-time data harvesting.
6. Import.io
Import.io is an online scraping tool that enables users to create datasets by importing data from specific web pages and exporting it to CSV. It's a no-code/low-code platform ideal for large enterprises seeking quick data extraction from websites. Import.io allows effortless scraping of millions of web pages without writing a single line of code. With APIs and webhooks, you can easily integrate data into applications. It offers both web-based and desktop tools for data extraction, making it adaptable to various user preferences.
Embrace Data-Driven Success
Data is no longer a luxury; it's a necessity across industries like healthcare and real estate. Predictive data analytics is on the rise, with businesses using data to forecast market trends and needs. Companies are recognizing the importance of data analytics for business insight, product development, and customer satisfaction. To thrive in this competitive landscape, choose a data scraper from our list or explore professional tools like those offered by Outsource BigData. With a range of data scraping solutions, you can effortlessly extract data from websites, even from those protected by bots. Visit the Outsource BigData website for more details on their data scraping products and services.
Original blog url: https://outsourcebigdata.com/blog/scraping-tool/6-top-scraping-tools-that-you-cannot-miss-in-2022/
0 notes
Text
Boost Your Retail Strategy with Quick Commerce Data Scraping in 2025
Introduction
The retail landscape is evolving rapidly, with Quick Commerce (Q-Commerce) driving instant deliveries across groceries, FMCG, and essential products. Platforms like Blinkit, Instacart, Getir, Gorillas, Swiggy Instamart, and Zapp dominate the space, offering ultra-fast deliveries. However, for retailers to stay competitive, optimize pricing, and track inventory, real-time data insights are crucial.
Quick Commerce Data Scraping has become a game-changer in 2025, enabling retailers to extract, analyze, and act on live market data. Retail Scrape, a leader in AI-powered data extraction, helps businesses track pricing trends, stock levels, promotions, and competitor strategies.
Why Quick Commerce Data Scraping is Essential for Retailers?
Optimize Pricing Strategies – Track real-time competitor prices & adjust dynamically.
Monitor Inventory Trends – Avoid overstocking or stockouts with demand forecasting.
Analyze Promotions & Discounts – Identify top deals & seasonal price drops.
Understand Consumer Behavior – Extract insights from customer reviews & preferences.
Improve Supply Chain Management – Align logistics with real-time demand analysis.
How Quick Commerce Data Scraping Enhances Retail Strategies?
1. Real-Time Competitor Price Monitoring
2. Inventory Optimization & Demand Forecasting
3. Tracking Promotions & Discounts
4. AI-Driven Consumer Behavior Analysis
Challenges in Quick Commerce Scraping & How to Overcome Them
Frequent Website Structure Changes Use AI-driven scrapers that automatically adapt to dynamic HTML structures and website updates.
Anti-Scraping Technologies (CAPTCHAs, Bot Detection, IP Bans) Deploy rotating proxies, headless browsers, and CAPTCHA-solving techniques to bypass restrictions.
Real-Time Price & Stock Changes Implement real-time web scraping APIs to fetch updated pricing, discounts, and inventory availability.
Geo-Restricted Content & Location-Based Offers Use geo-targeted proxies and VPNs to access region-specific data and ensure accuracy.
High Request Volume Leading to Bans Optimize request intervals, use distributed scraping, and implement smart throttling to prevent getting blocked.
Unstructured Data & Parsing Complexities Utilize AI-based data parsing tools to convert raw HTML into structured formats like JSON, CSV, or databases.
Multiple Platforms with Different Data Formats Standardize data collection from apps, websites, and APIs into a unified format for seamless analysis.
Industries Benefiting from Quick Commerce Data Scraping
1. eCommerce & Online Retailers
2. FMCG & Grocery Brands
3. Market Research & Analytics Firms
4. Logistics & Supply Chain Companies
How Retail Scrape Can Help Businesses in 2025
Retail Scrape provides customized Quick Commerce Data Scraping Services to help businesses gain actionable insights. Our solutions include:
Automated Web & Mobile App Scraping for Q-Commerce Data.
Competitor Price & Inventory Tracking with AI-Powered Analysis.
Real-Time Data Extraction with API Integration.
Custom Dashboards for Data Visualization & Predictive Insights.
Conclusion
In 2025, Quick Commerce Data Scraping is an essential tool for retailers looking to optimize pricing, track inventory, and gain competitive intelligence. With platforms like Blinkit, Getir, Instacart, and Swiggy Instamart shaping the future of instant commerce, data-driven strategies are the key to success.
Retail Scrape’s AI-powered solutions help businesses extract, analyze, and leverage real-time pricing, stock, and consumer insights for maximum profitability.
Want to enhance your retail strategy with real-time Q-Commerce insights? Contact Retail Scrape today!
Read more >>https://www.retailscrape.com/fnac-data-scraping-retail-market-intelligence.php
officially published by https://www.retailscrape.com/.
#QuickCommerceDataScraping#RealTimeDataExtraction#AIPoweredDataExtraction#RealTimeCompetitorPriceMonitoring#MobileAppScraping#QCommerceData#QCommerceInsights#BlinkitDataScraping#RealTimeQCommerceInsights#RetailScrape#EcommerceAnalytics#InstantDeliveryData#OnDemandCommerceData#QuickCommerceTrends
0 notes
Text
How to Leveraging Mobile App Scraping for Retail Success?

Mobile apps have become a vital consumer shopping channel in today's post-pandemic world. With smartphone users spending an average of over 3 hours on their phones daily, it's clear that mobile apps are increasingly preferred over traditional eCommerce apps. Recognizing this shift, Mobile App Scraping has introduced an innovative mobile app scraping solution to complement its successful eCommerce app scraping technology. This advanced solution is designed to help businesses thrive in the evolving retail landscape.
Let's dive deeper into the process and technology behind mobile app scraping, which can be approached in two ways:

Scenario 1:
When composite APIs are open (e.g., Amazon) - In such cases, the scraping process is similar to standard app scraping, with some initial setup required. Mobile App Scraping can utilize the available APIs of platforms like Amazon to access and extract the desired data. The process involves making API requests, handling responses, and parsing the retrieved data.
Scenario 2:
Whenever composite APIs get encrypted (e.g., Dollar General, HEB, Target, Stop & Shop, etc.) - This scenario presents a more complex challenge. Mobile App Scraping employs specialized techniques such as mobile device extraction, OCR (Optical Character Recognition), as well as other machine learning methods to overcome the encryption and extract data from these apps. Mobile App Scraping leverages advanced technologies to navigate the encrypted APIs and interfaces, ensuring accurate and reliable data extraction.
In both scenarios, Mobile App Scraping utilizes its expertise and advanced methodologies to scrape mobile apps efficiently and effectively, providing businesses with valuable data insights to enhance their operations and decision-making processes.
Methodology
Step 1: Recording a mobile session/navigation using unique visual navigation scrapers Technology consideration
Mobile App Scraping employs a virtual device cloud infrastructure to ensure scalability and efficiency. It utilizes an emulated cluster of devices that connect through an intelligent proxy network.
In the first step of the methodology, special visual navigation scrapers are utilized to record mobile sessions and navigation. These scrapers interact with the mobile app interface, mimicking user actions and capturing the relevant data. This approach allows Mobile App Scraping to navigate the app, access different screens, and interact with various elements to gather the desired information.
To achieve scalability, a virtual device cloud infrastructure is employed. This infrastructure emulates a cluster of devices, ensuring that the scraping process can be performed at scale. Additionally, an intelligent proxy network handles communication between the devices and the app, ensuring efficient data retrieval.
By combining special visual navigation scrapers, an actual device cloud, and an intelligent proxy network, Mobile App Scraping ensures a robust and scalable approach to recording mobile sessions and navigating through mobile apps for data scraping purposes.

Step 2: Identify product ROI (Region of Interest)
To accurately scrape text for each product, Mobile App Scraping utilizes an Object Detection algorithm to identify the ROI of individual products within a frame, regardless of the number of products present.
The input files are passed through a custom fine-tuned version of the YOLOv5 (You Only Look Once) architecture, which excels in fast and accurate inference. YOLOv5 can swiftly detect and localize each product's ROI within the frame.
Since a video typically consists of multiple frames per second, the exact product ROIs are possible. To address this, a deduplication stage is introduced. This stage eliminates identical product ROIs, allowing faster and more efficient data processing. The resulting ROIs from the video frames are then cached as images, ready for further analysis and extraction.
By utilizing the Object Detection capabilities of YOLOv5 and implementing deduplication techniques, Mobile App Scraping ensures the accurate identification and localization of product ROIs, laying the foundation for subsequent data processing and extraction.

Step 3: Identify product components
In this stage, Mobile App Scraping focuses on identifying specific components of the products, such as price, product information, product image, and more. The same components to be identified may vary based on the specific mobile app being scraped.
To achieve precise component identification, Mobile App Scraping applies YOLOv5 again, but with a different instance or configuration compared to Step 2. This allows for more granular consideration in detecting and localizing the desired components within the product ROIs.
By leveraging YOLOv5's capabilities, Mobile App Scraping ensures accurate and reliable identification of various product components, enabling the extraction of crucial information such as prices, descriptions, and images. This stage is critical in gathering the specific data elements needed for further analysis and utilization.

Step 4: Extract components with OCR
In this stage, Mobile App Scraping completes the textual extraction process now that all details of the products have been identified. A custom-trained OCR (Optical Character Recognition) framework is deployed to achieve this.
The OCR framework is specifically trained to recognize and extract text from the identified components of the products. It utilizes advanced algorithms and machine learning techniques to accurately extract textual information such as product names, prices, descriptions, and other relevant details.
Mobile App Scraping ensures precise and reliable textual data extraction from the identified product components by deploying a custom-trained OCR framework. This stage is crucial in capturing the detailed information needed for further analysis, processing, or integration with other systems.

Step 5: Accessing the final output
In the final stage, the text extraction output and relevant metadata are stored in a database. Mobile App Scraping applies unit tests and quality checks to ensure the accuracy and integrity of the extracted data.
Once the data is verified and validated, it is transformed into the desired format suitable for the client's needs. This transformation process ensures the information is structured appropriately and ready to be accessed, sent, uploaded, or requested via API (Application Programming Interface).
By storing the data in a database and applying rigorous quality checks, Mobile App Scraping guarantees the reliability and usability of the extracted information. The transformed data can now be seamlessly integrated into the client's systems or applications, empowering them with valuable insights from mobile app scraping.

Conclusion
In conclusion, as the retail landscape evolves with the rise of mobile and social commerce and the emergence of the metaverse, the need for advanced AI and analytics becomes paramount. Mobile App Scraping recognizes this need and is dedicated to providing retailers and brands with sophisticated and accurate analytics across the retail ecosystem. They have developed an cutting-edge mobile app scraping solution to cater to the evolving needs of the retail industry.
Moreover, Mobile App Scraping has extended its proprietary technology to the metaverse, enabling the scraping of retail stores in virtual platforms like Decentraland, Roblox, Sandbox, Meta, and more. This expansion reflects their commitment to staying at the forefront of technological advancements and ensuring that retailers have access to critical data and insights across multiple platforms.
With the growing popularity of mobile commerce and the increasing competition in the retail space, mobile app scraping has become essential for maintaining competitive prices, assortments, and digital shelf ranking. By leveraging Mobile App Scraping's cutting-edge solutions, retailers can stay ahead of the curve and make informed decisions based on accurate and real-time data.
In summary, Mobile App Scraping offers innovative mobile app scraping solutions alongside their established eCommerce app scraping technology, empowering retailers to thrive in the dynamic retail landscape and meet the demands of modern consumers.
Source: https://www.mobileappscraping.com/mobile-app-scraping-for-retail-success.php
#mobileappscrapingforretail#mobileappscrapingforeCommerce#eCommercemobileappscraping#retailmobileappscraping
0 notes
Text
Beyond the Basics: Elevating Your Ecommerce App Development Strategy
Would you like to give your online business an upper hand? Provided that this is true, fostering a portable application is an incredible approach. Fostering an application for your web based business can be an incredible method for arriving at additional clients, increment deals, and proposition a superior client experience. Yet, essentially making a portable application isn't sufficient. To really succeed, it's essential to create an application that isn't simply utilitarian and simple to utilize, yet in addition connecting with and outwardly engaging. In this blog entry, we'll talk about the critical elements to consider while fostering an online business versatile application, and how to go past the nuts and bolts to make an application that stands apart from the opposition.
Visit also: Android app development company in Dubai
Key Variables to Consider
While fostering a web based business portable application, there are a few vital variables to consider that can enormously influence its prosperity. As a matter of some importance, understanding your ideal interest group and their preferences is pivotal. Lead exhaustive statistical surveying to acquire experiences into their socioeconomics, interests, and shopping propensities. This data will direct you in making an application that takes care of their particular requirements and wants.
Another significant variable is client experience (UX). A consistent and natural UX is fundamental for keeping clients drew in and fulfilled. Think about variables like simple route, clear item portrayals, and a smoothed out checkout process. Consolidating highlights like item suggestions, customized warnings, and client surveys can additionally upgrade the UX and energize rehash buys.
Moreover, it's significant to improve your application for various cell phones and working frameworks. Lead careful testing to guarantee similarity and responsiveness across different stages. Moreover, stay aware of the furthest down the line mechanical headways to guarantee your application stays important and bleeding edge.
Ultimately, consider integrating social sharing and reference highlights to increment brand perceivability and empower verbal exchange promoting. Incorporate virtual entertainment stages to permit clients to impart items and advancements to their organizations, accordingly extending your scope.
Via cautiously considering these key elements, you can create an online business portable application that meets the fundamental necessities as well as gives an extraordinary and drawing in experience for your clients, at last prompting expanded deals and an upper hand on the lookout.
Execution Improvement
With regards to fostering an online business portable application, execution improvement is a basic viewpoint that ought not be disregarded. A sluggish stacking application or one that oftentimes crashes can rapidly disappoint clients and lead to high bob rates. To guarantee your application moves along as expected and proficiently, there are a few presentation improvement methodologies to consider.
In the first place, upgrade your application's code and decrease its size to further develop stacking times. This should be possible by minifying and compacting code, as well as eliminating any pointless components or functionalities. Also, influence reserving strategies to store every now and again got to information, decreasing the requirement for dull information recovery and working on by and large execution.
One more key figure execution improvement is picture advancement. Huge picture scrapes can fundamentally dial back an application's stacking speed. To moderate this, pack and resize pictures without undermining their quality. Consider utilizing responsive picture procedures to serve the most suitable picture size for every client's gadget.
Moreover, carrying out effective information synchronization procedures is urgent for improving application execution. Utilize nonconcurrent information stacking and getting strategies to forestall delays and keep a consistent client experience. Consider using foundation cycles or message pop-ups to refresh application content without requiring the client to physically invigorate the application.
Ultimately, ordinary observing and testing of your application's exhibition is fundamental. Recognize and determine any bottlenecks or execution issues expeditiously. Monitor key execution markers (KPIs, for example, application reaction time, mistake rates, and client input to advance and upgrade your application's exhibition consistently.
By zeroing in on execution improvement, you can make a web based business versatile application that gives a quick and consistent client experience, prompting higher consumer loyalty and expanded changes.
For more info, contact: [email protected]
People can also read: Mobile app development companies in Abu Dhabi
0 notes
Text
Large-Scale Web Scraping: An Ultimate Guide

The Internet is a vast place. There are billions of users who produce immeasurable amounts of data daily. Retrieving this data requires a great deal of time and resources.
To make sense of all that information, we need a way to organize it into something meaningful. That is where large-scale web scraping comes to the rescue. It is a process that involves gathering data from websites, particularly those with large amounts of data.
What Is Large-Scale Web Scraping?
Large Scale Web Scraping is scraping web pages and extracting data from them. This can be done manually or with automated tools. The extracted data can then be used to build charts and graphs, create reports and perform other analyses on the data.

Large Scale Web Scraping is an essential tool for businesses as it allows them to analyze their audience's behavior on different websites and compare which performs better.
3 Major Challenges In Large Scale Web Scraping
1. Performance
Performance is one of the significant challenges in large-scale web scraping.
The main reason for this is the size of web pages and the number of links resulting from the increased use of AJAX technology. This makes it difficult to scrape data from many web pages accurately and quickly.
2. Web Structure
Web structure is the most crucial challenge in scraping. The structure of a web page is complex, and it is hard to extract information from it automatically. This problem can be solved using a web crawler explicitly developed for this task.
3. Anti-Scraping Technique
Another major challenge that comes when you want to scrape the website at a large scale is anti-scraping. It is a method of blocking the scraping script from accessing the site.
If a site's server detects that it has been accessed from an external source, it will respond by blocking access to that external source and preventing scraping scripts from accessing it.
Read More ......
#Large scale web scraping#web scraping#data scraping services#data extraction#eCommerce datascraping#Mobile app Scraping
1 note
·
View note